Industrial molds, or toolings, play a vital role in discrete manufacturing, responsible for shaping virtually every product and component in all types of industries, from automotive and electronics to medical appliances and packaging. Unlike equipment and machinery, molds are often treated as consumables due to their lower cost and shorter lifespan. Yet these same factors make them more demanding in terms of maintenance and replenishment. In fact, mold lifecycle management plays a make-or-break role in successful manufacturing operations. This article examines the difficulties manufacturers face in managing mold lifecycle, and how machine learning, analytics, and AI can be applied to improve this process.
Current Challenges in Mold Lifecycle Management
Location tracking. One of the most challenging aspects of mold lifecycle management is tracking mold location. To avoid production downtime, suppliers often request replacement molds from OEMs before the active ones reach end-of-life. These new molds are typically stored in their warehouses, taking up significant storage space. Currently, most suppliers manually keep track of mold location on spreadsheets. With some suppliers managing dozens to hundreds of molds simultaneously, it is very easy to lose track of record, resulting in lost and misplaced molds. Since OEMs rely on these spreadsheets to track their mold expenditure and inventory, their calculations end up inaccurate and inconsistent.
Cycle count tracking. Each type of mold has a unique life expectancy. Some molds only operate up to 500 cycles, while others can last over 1 million cycles. To ensure timely replacement or refurbishment, suppliers must closely track each mold’s cycle count. Yet, this data is also recorded manually in spreadsheets, which are prone to errors and delays. When OEMs rely on these spreadsheets for mold replenishment and maintenance decisions, the risk of late maintenance and quality issues increases significantly.
Manual auditing. Without a reliable cycle count tracking mechanism, OEMs often resort to periodic on-site audits to monitor mold inventory and lifespan. This approach is both labour-intensive and time-consuming, and it frequently leads to discrepancies and disputes between OEMs and suppliers, damaging their long-term relationships.
Reactive maintenance. Under the current manual tracking system, mold maintenance is often implemented after production issues surface. This results in a high volume of defective parts that end up scrapped. Even more concerning, continuing to run molds beyond their intended maintenance point can cause irreversible damage, shortening their lifespan and increasing replacement costs.
Digitalizing Mold Lifecycle Management
Rather than relying on manual tracking and reporting, eMoldino’s digital tooling asset management solution automates the entire process. By equipping each mold with industrial IoT sensors that enable real-time data collection, it eliminates the need for error-prone spreadsheets and manual updates.
Automated location tracking. The GPS embedded in the sensors automatically reports the real-time location of each mold, giving both OEMs and suppliers full visibility into mold inventory across the supply chain. This enables accurate tracking of mold quantity and location without the need for manual audits, and also ensures data continuity before and after location changes.
Cycle count tracking. The sensor counts the number of times a mold opens and closes, recording this as a full cycle. It also captures the duration of each shot to calculate cycle time. In addition to these metrics, the sensor also tracks important measures such as temperature, uptime, and pressure, all of which are important in determining the life expectancy of the mold. All this data is transmitted in real-time to a centralized control center managed by the OEM and shared with suppliers, enabling them to collaborate on mold ordering, replacement, and maintenance.
The Role of AI and Analytics in Mold Lifecycle Management
As noted earlier, reactive mold maintenance is essentially too late. By the time defective parts are identified, the mold has already sustained significant damage, requiring costly repairs or even replacement. Furthermore, reactive maintenance often leads to shortened mold lifespan, unexpected downtime, and a high scrap rate, all of which add to manufacturing cost and capital expenditure.
From reactive to predictive maintenance. With real-time mold data now readily available, a wide array of automation opportunities emerge. eMoldino leverages machine learning to analyze patterns and trends in its collected data, enabling an accurate prediction of each mold’s maintenance schedule. This AI-driven approach not only optimizes maintenance timing but also reduces downtime and extends the overall mold lifespan.
Automated report generation. With the help of AI, manual reporting through spreadsheets and emails becomes a thing of the past. eMoldino’s GenAI engine automatically visualizes data and their analyses using intuitive graphs and charts, then creates clear, actionable reports for both OEMs and suppliers. This eliminates the need for manual data entry and reporting, allowing manufacturers to stay focused on what they do the best – manufacturing.
About the author
eMoldino
eMoldino aims to digitalize, streamline, and transform your manufacturing and supply chain operations. We help global manufacturers who want to drive corporate innovation while maintaining the core values of collaboration and sustainability. Talk with us to learn more →
Did you enjoy reading this article?
0 / 5. Vote count: 0
Explore Our Latest Posts
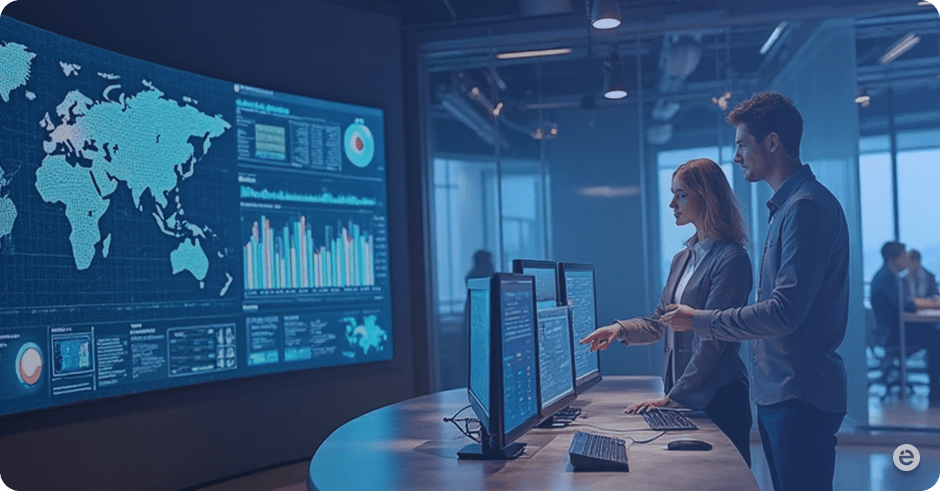
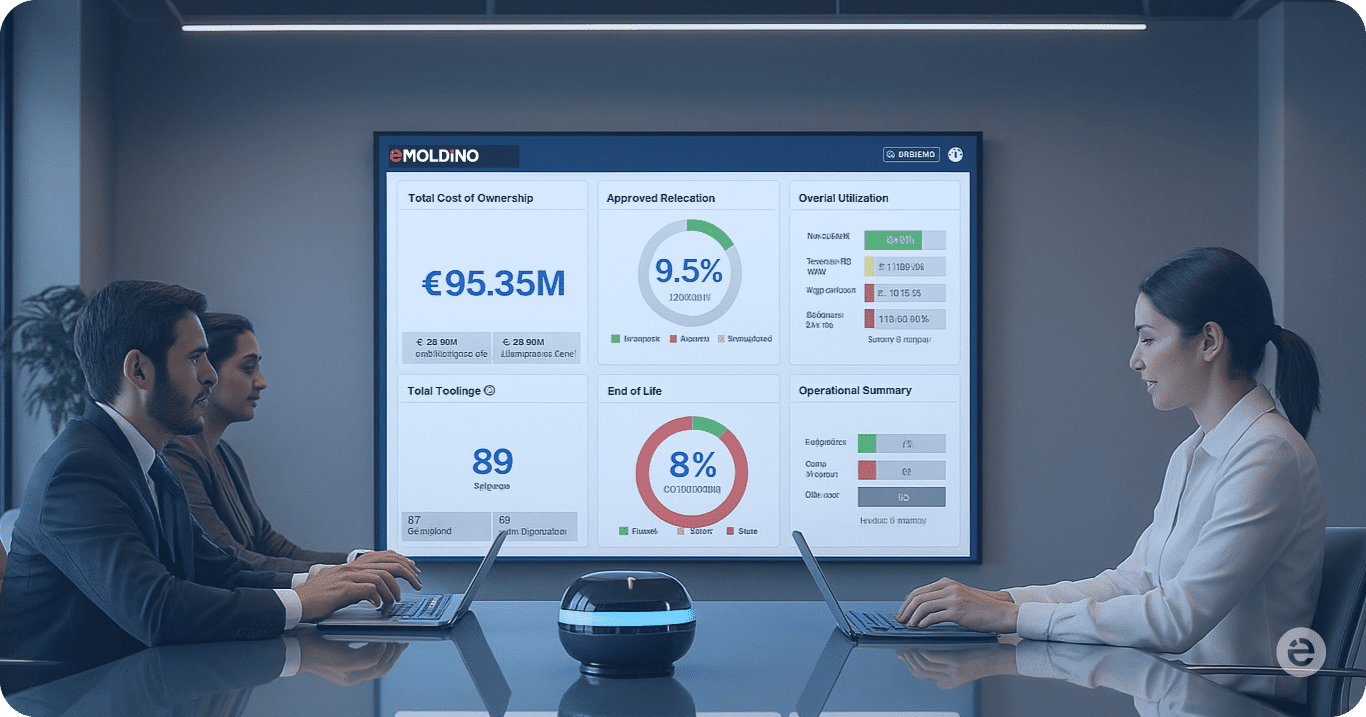
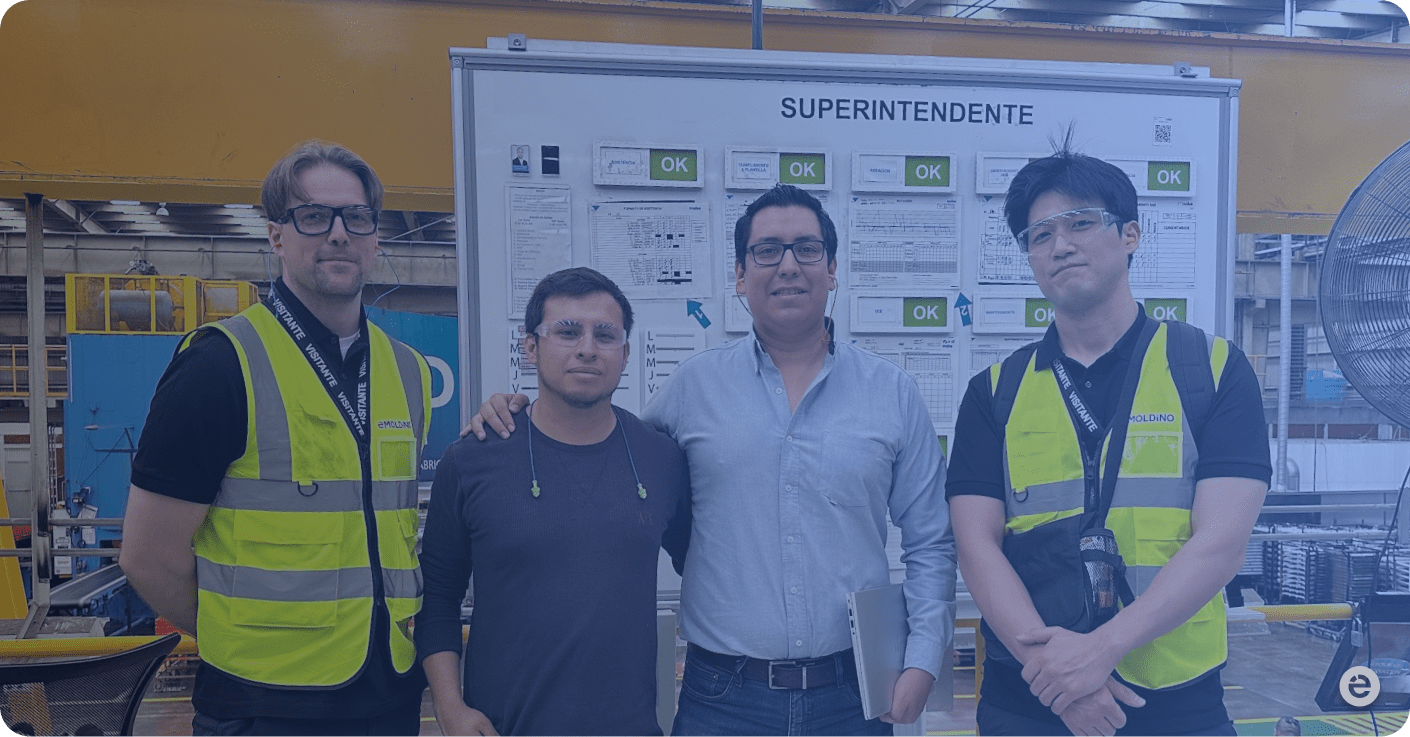
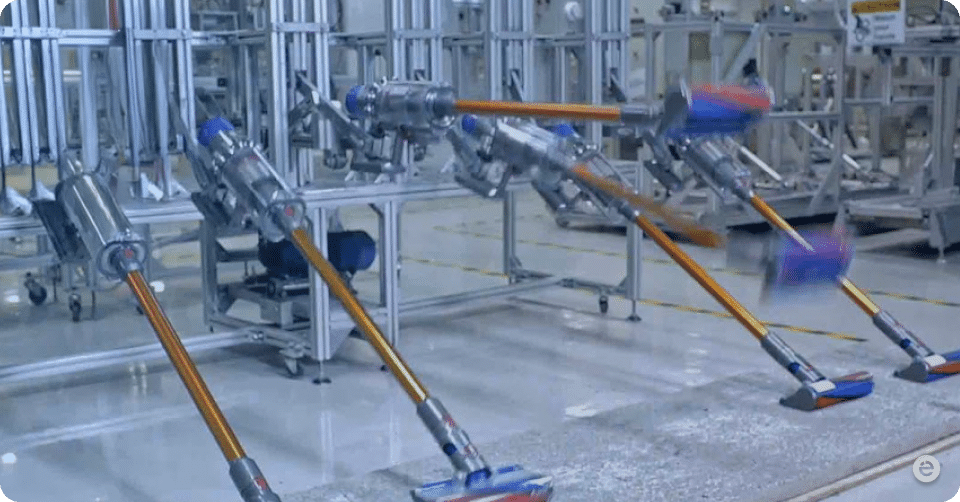
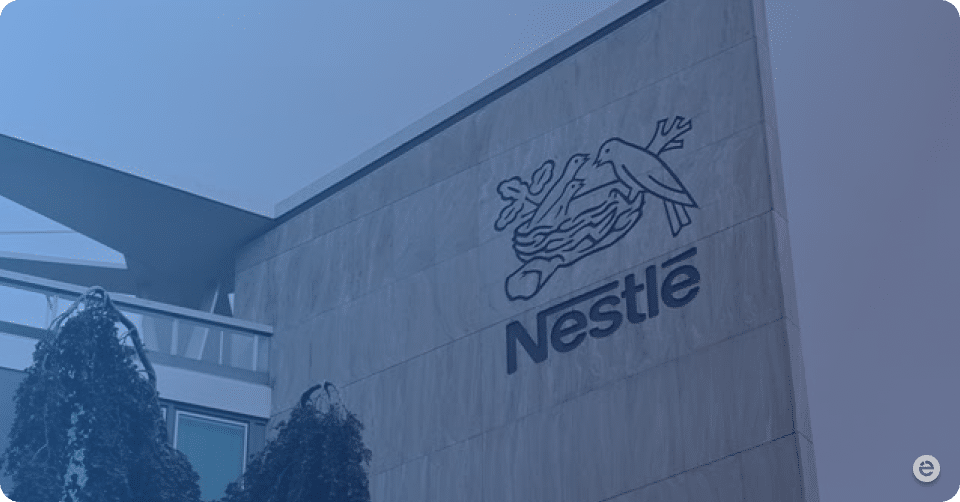
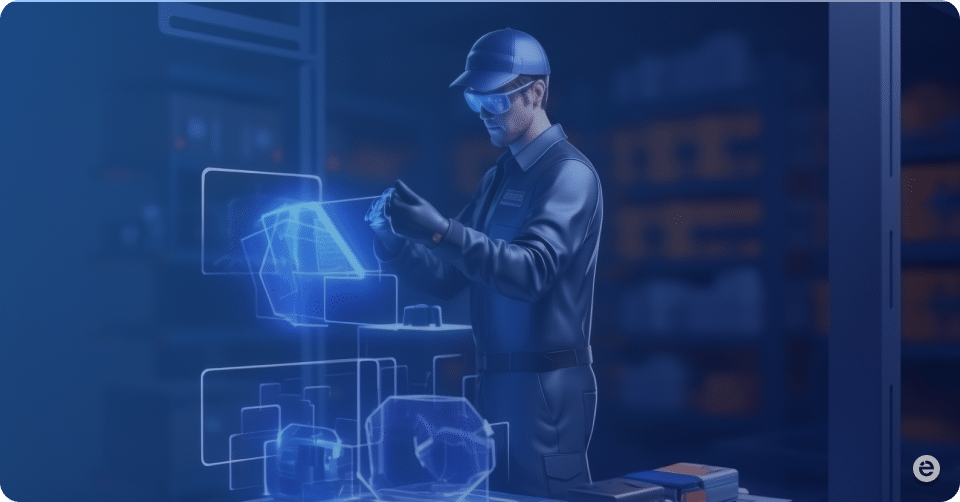
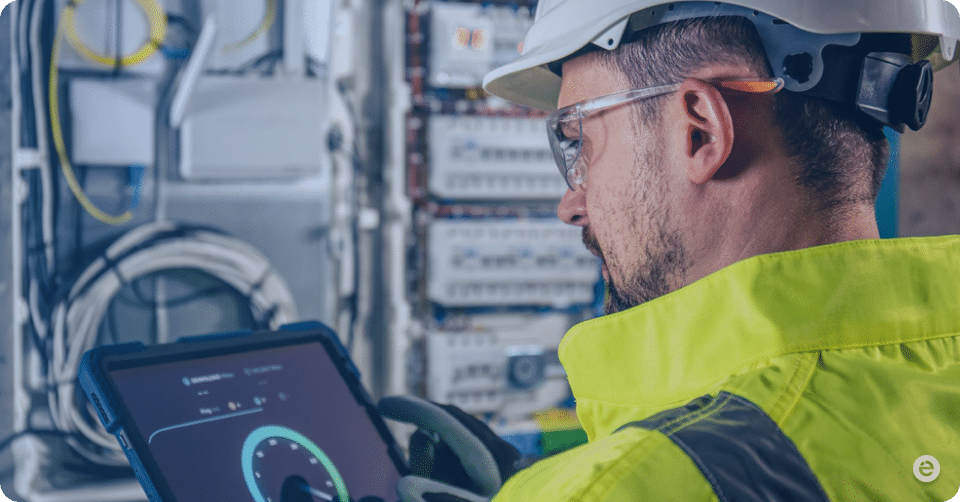
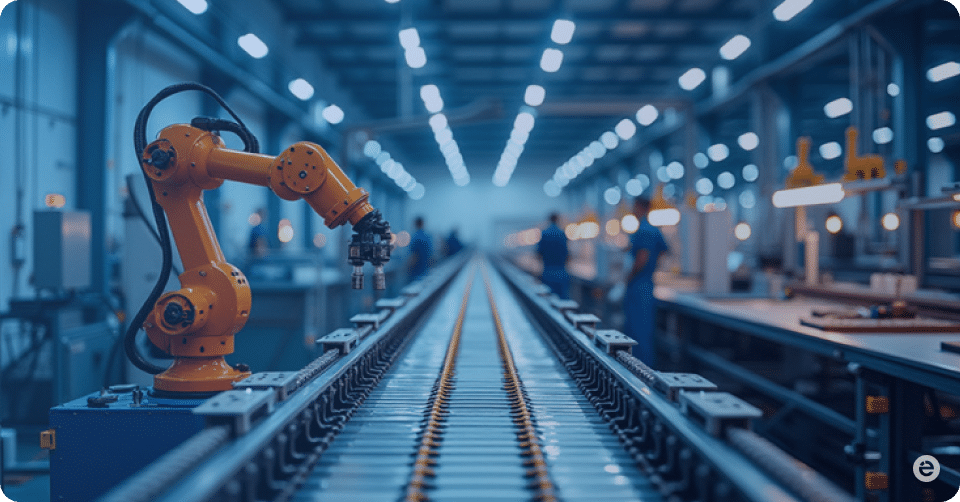
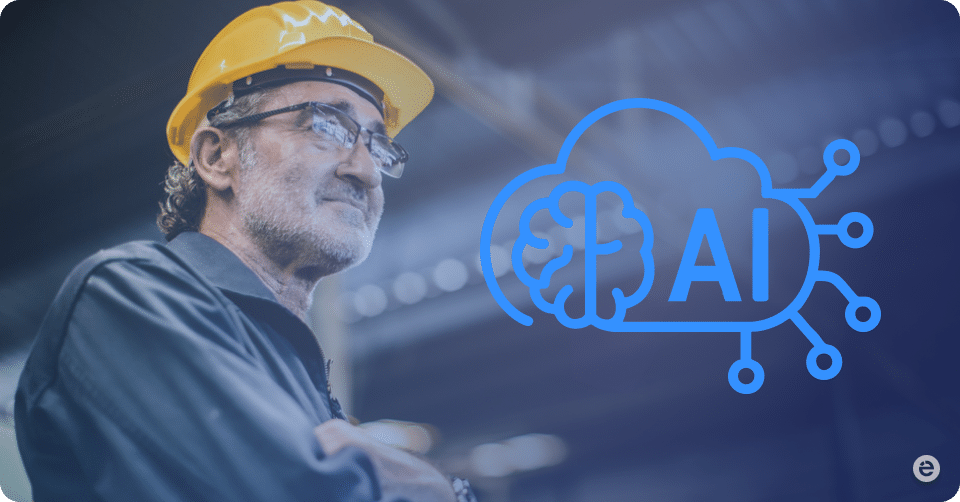
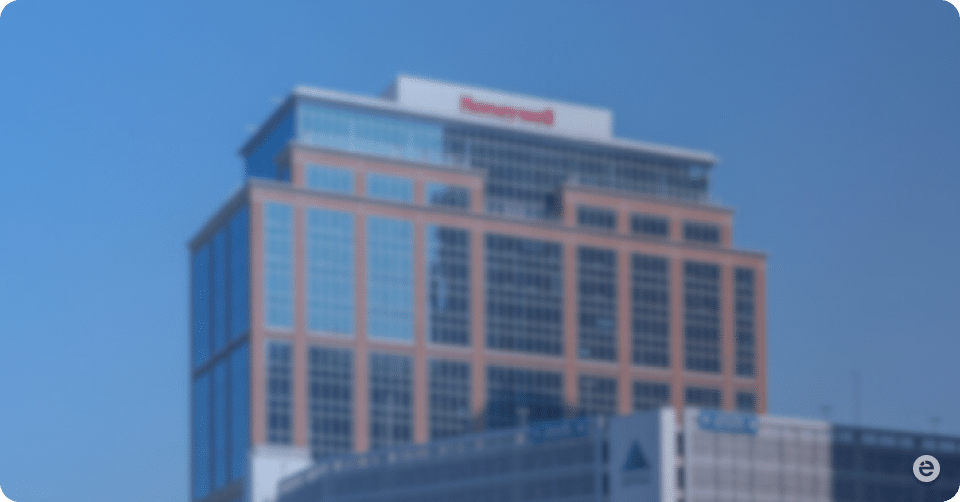
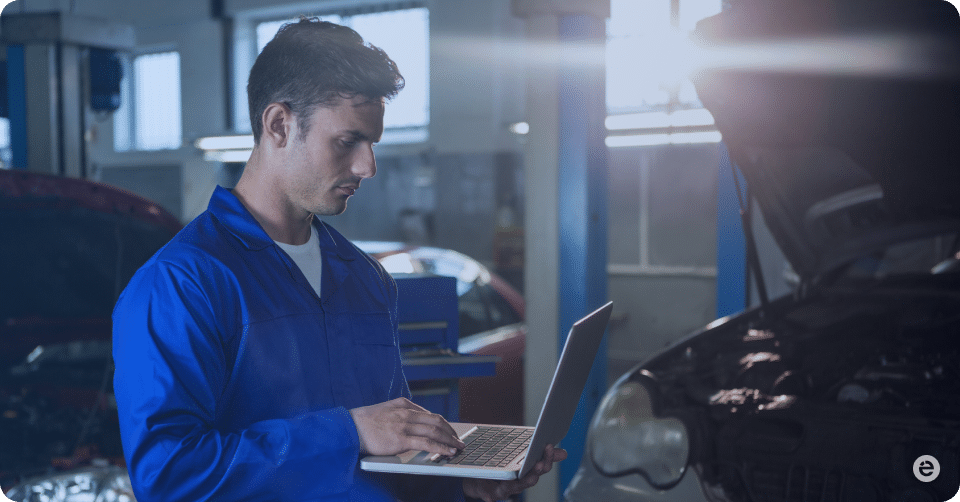
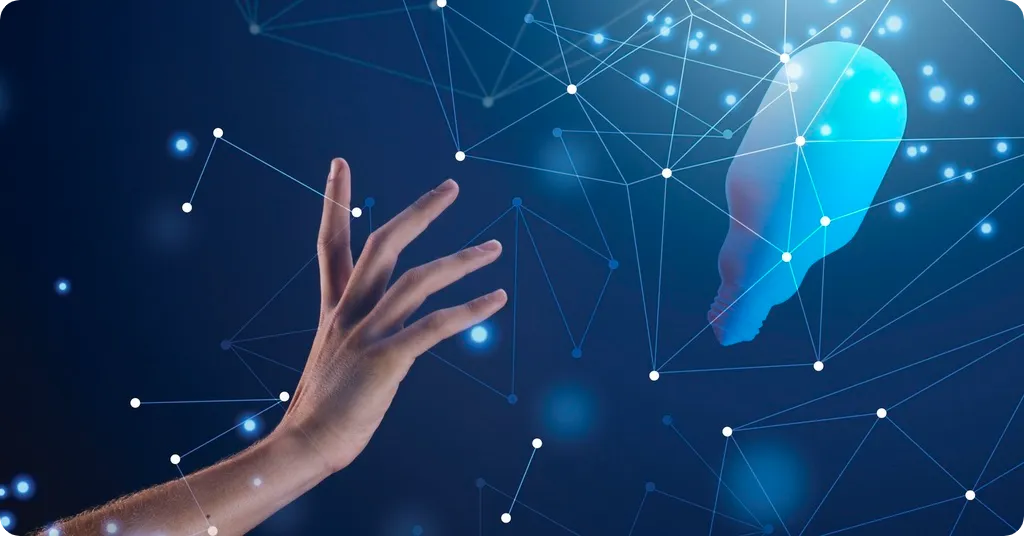
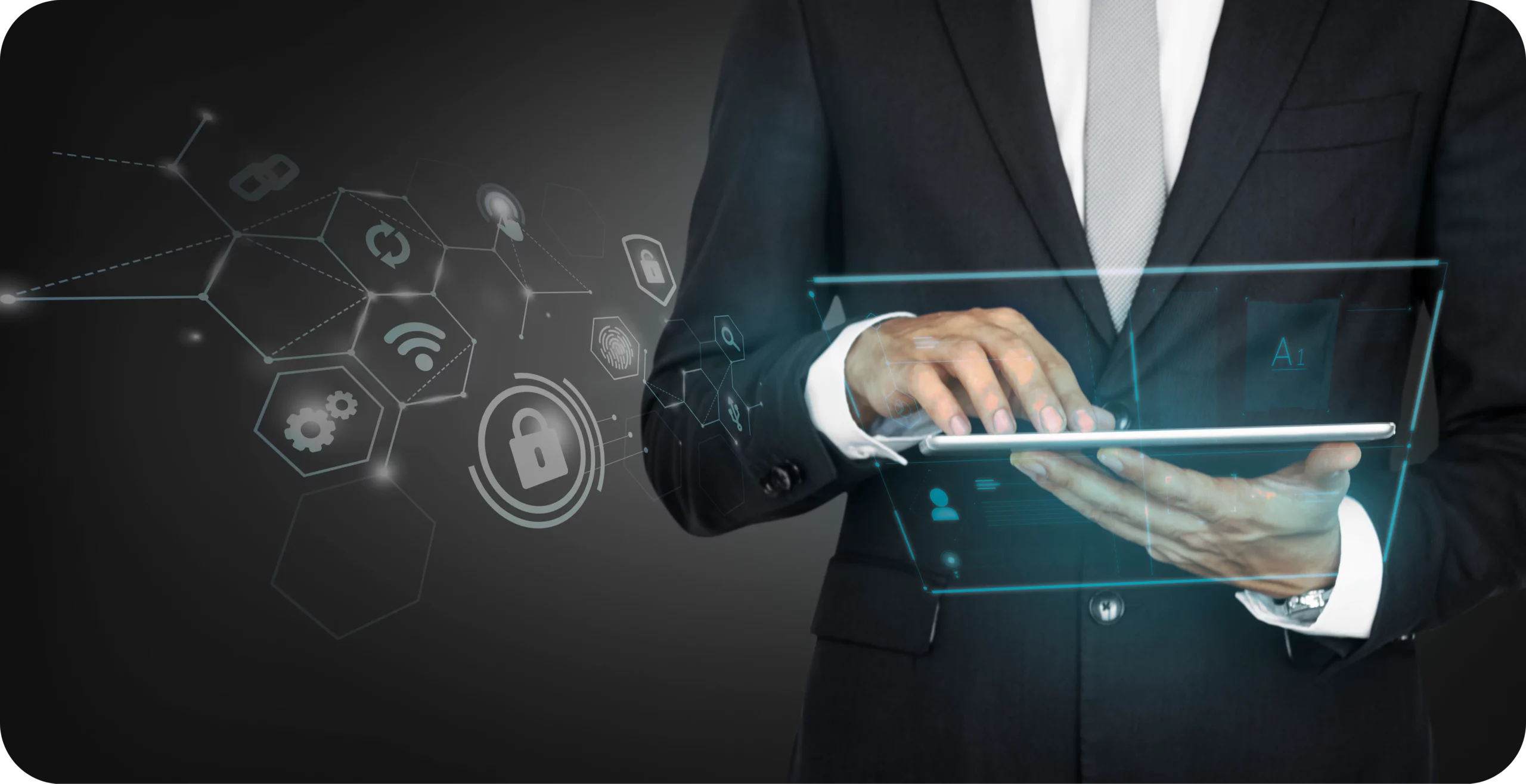
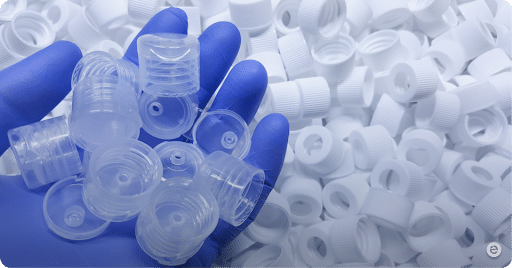
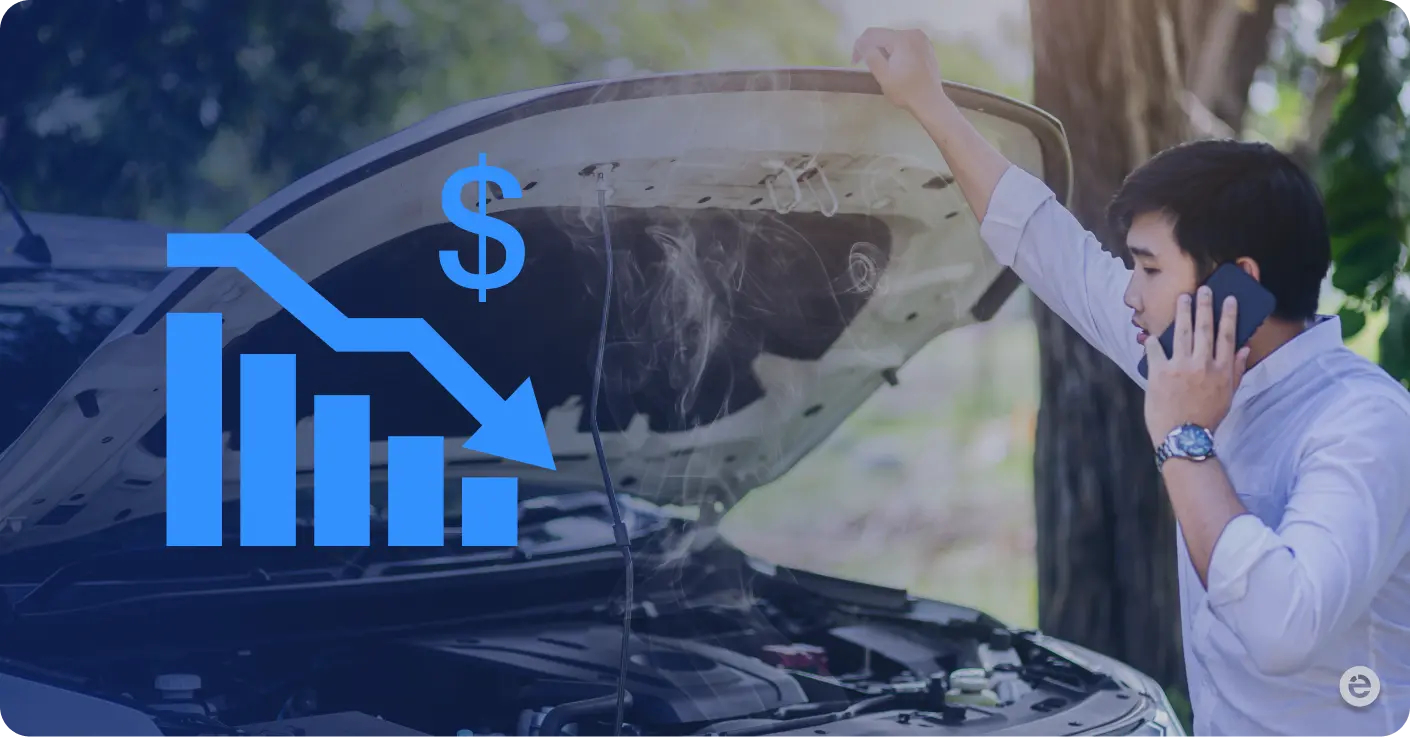